PROFL: A Privacy-Preserving Federated Learning Method with Stringent Defense Against Poisoning Attacks
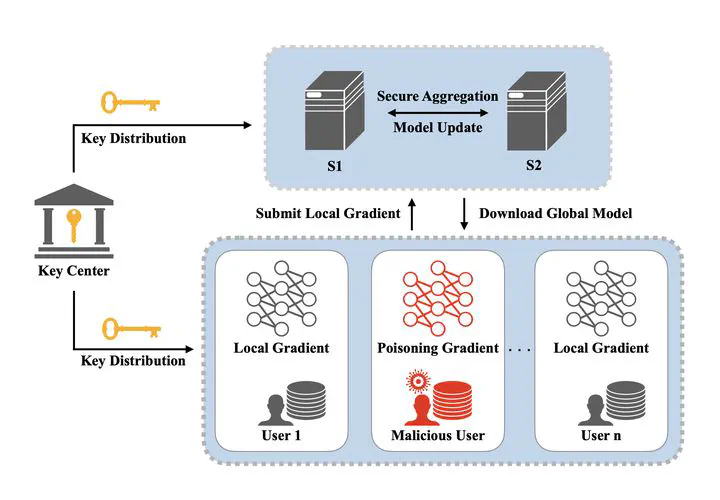
Abstract
Federated Learning (FL) faces two major issues, privacy leakage and poisoning attacks, which may seriously undermine the reliability and security of the system. Overcoming them simultaneously poses a great challenge. This is because pri- vacy protection policies prohibit access to users’ local gradients to avoid privacy leakage, while Byzantine-robust methods necessi- tate access to these gradients to defend against poisoning attacks. To address these problems, we propose a novel privacy-preserving Byzantine-robust FL framework PROFL. PROFL is based on the two-trapdoor additional homomorphic encryption algorithm and blinding techniques to ensure the data privacy of the entire FL process. During the defense process, PROFL first utilize secure Multi-Krum algorithm to remove malicious gradients at the user level. Then, according to the Pauta criterion, we innovatively propose a statistic-based privacy-preserving defense algorithm to eliminate outlier interference at the feature level and resist impersonation poisoning attacks with stronger concealment. De- tailed theoretical analysis proves the security and efficiency of the proposed method. We conducted extensive experiments on two benchmark datasets, and PROFL improved accuracy by 39% to 75% across different attack settings compared to similar privacy-preserving robust methods, demonstrating its significant advantage in robustness.
Type
Publication
In 2024 27th International Conference on Computer Supported Cooperative Work in Design